FAB: A Robust Facial Landmark Detection Framework for Motion-Blurred Videos
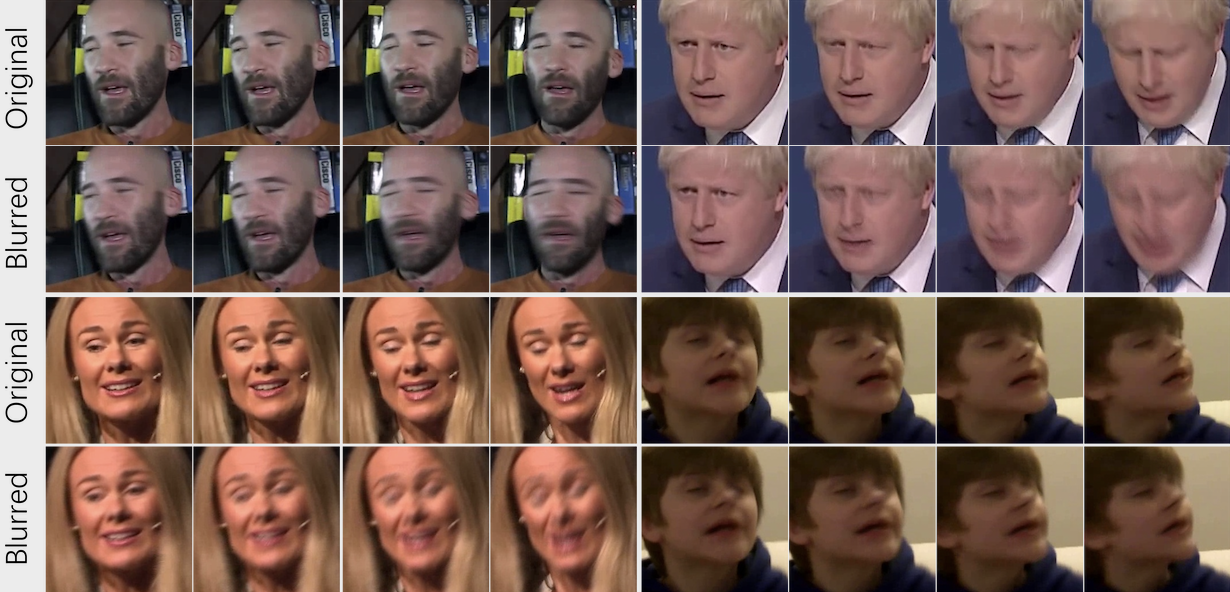
Abstract
In order to generate more severe motion blur, larger motion in original videos is a need. We select subsets (31 videos in the training set and 9 videos in the testing set) from 300VW with large motion according to a face motion intensity index. The face motion intensity is defined by accumulating the movement of the left eye during a time unit and normalizing it with the inter-ocular distance. As is shown in Figure above, the selected videos are characterized with far more severe motion intensity. For each adjacent three frames, we interpolated them with 20 subframes according to the optical flow. The mean value of these 20 subframes is calculated to mimic motion blur. Annotation of each generated frame is taken from the middle-time subframe. The blurred-300VW dataset contains obvious motion blur especially when the face moves greatly, which is suitable for the illustration of this work.
Citation
@inproceedings{keqiang2019fab, author = {Sun, Keqiang and Wu, Wayne and Liu, Tinghao and Yang, Shuo and Wang, Quan and Zhou, Qiang and and Ye, Zuochang and Qian, Chen}, title = {FAB: A Robust Facial Landmark Detection Framework for Motion-Blurred Videos}, booktitle = {ICCV}, month = October, year = {2019} }